INTRODUCTION
In 2012, we made a groundbreaking achievement by introducing the use of microphone arrays into long-term noise monitoring to study the effects of environmental and industrial noise on humans. Our work was the result of the ARRS Research Program P2-0354: Noise Control and its Effects on People. We reconfigured microphone arrays to identify the dominant noise sources in real-time, by detecting the direction of sound sources in polar coordinates on horizontal plane. In the SANSIC project (System for Automatic Noise Source Identification and Classification), we developed algorithms that required only four microphones. Methodology was patented in 2015. In 2018 we introduced a new parameter for describing complex acoustic environments, called the Immission Directivity. This parameter enabled spatial filtering of sound events, allowing for the extraction of spatial features of sound events. We then combined the spatial features extracted from the Immission Directivity with psychoacoustic features of sound events, for implementation in Artificial Intelligence (AI) algorithms (K-means, Self-Organizing Maps, and ANN).
Project proposes a methodology based on a network of IoT microphone arrays, which will allow localization of each sound event in every 50 milliseconds, and its classification. The methodology will enable tracking and counting of selected species producing sounds within a designated space. It will also allow for counting the number of different animals producing sound within the observed area. The proposed methodology is therefore a game-changer for monitoring biodiversity and for noise mapping in urban environments. Concept of “Advanced Sound Maps” is shown in Fig 1.
Fig. 1. Concept of Advanced Sound Maps based on the network of microphone arrays, left) in the natural environment to assess biodiversity and right) proof of concept in an urban/industrial environment for the purpose of noise abatement planning.
Excessive noise pollution from traffic is the second most important environmental cause of health problems in Europe, with an annual cost of over 2.7 billion euros. The EU has adopted the EU Action Plan: “Towards a Zero Pollution for Air, Water and Soil” as part of the European Green Deal to reduce the proportion of people chronically disturbed by traffic noise by 30%. Simultaneously, the decline in biodiversity has become a critical issue as species and populations disappear from ecosystems at the rate of a mass extinction event. Biodiversity provides numerous ecosystem services that are essential for human well-being and survival, making the conservation of biodiversity critical. The EU has developed the 2030 Biodiversity Strategy to protect and restore habitats, conserve species and ecosystems, and ensure that biodiversity is integrated into all sectors and activities.
The proposed methodology for noise monitoring addresses both issues. Advanced Sound Maps (ASM) with identified noise sources can be synthesized from data obtained from a network of IoT microphone arrays measuring Immission Directivity, enabling efficient control of noise pollution in urban areas. By combining the Immission Directivity” with psychoacoustic features of sound events, AI algorithms can be used to classify different sound sources, providing valuable information to policy makers to identify and address specific noise sources and their impact on human health and well-being. The proposed methodology has the potential to make a significant contribution to addressing urban noise pollution, a growing problem in many cities worldwide.
In addition, effective biodiversity monitoring tools are critical to the success of biodiversity conservation. Automated sensors for remote monitoring, combined with machine learning classification methods, are effective in identifying species in images or recordings. Acoustic monitoring is a promising approach to biodiversity monitoring, given that many animals use sound signals for communication. This method is cost-effective, requires fewer personnel, and can be conducted without human presence, making it a valuable tool for monitoring animal presence over time.
Despite the significant impact of noise on the environment, biodiversity, and quality of life, no research projects on this topic have been funded in Slovenia in the last 8 years. The proposed project has the potential to make a significant contribution to addressing noise pollution and biodiversity and is consistent with EU environmental objectives. We believe that this project is essential for the progress of environmental research in Slovenia and should be approved for funding.
OBJECTIVES OF THE PROPOSED RESEARCH PROJECT
This interdisciplinary research project aims to build on prior research achievements in the field of noise measurement methods, noise mapping, identification and classification of noise events, and the reduction of their impact on humans. By achieving three objectives, the project supports the EU’s goal of achieving zero air pollution as outlined in relevant documents.
Objective 1: Develop and implement a new method for real-time localization and classification of sound events using a network of IoT microphone arrays and Immission Directivity. This will enable the creation of new types of sound event maps for both urban and natural environments, supporting the EU and Slovenian initiatives for creating DIGITAL TWINS.
Objective 2: Develop and test a new method for monitoring biodiversity in natural environments using a network of IoT microphone arrays, which will be capable of precise localization of individuals in the field.
Objective 3: Provide a new methodology for data mining from the network of IoT microphone arrays, which will lead to more efficient and cost-effective noise abatement. This will help to reduce noise annoyance, sleep disorders, and health risks, supporting the EU’s Zero pollution action plan.
The project’s objectives will be accomplished through research efforts that involve proving four hypotheses.
Hypothesis 1: “An advanced sound map” indicating the location of the sound source and its class/type can be synthesized when features of the sound event detected by the IoT microphone array and the parameter Immission directivity, are extracted simultaneously in real time and recorded. The location can be calculated using advanced triangulation methods or inverse methods. Classification can be done by a combination of supervised classification for spatial sound event features and unsupervised, self-growing classification for sound signal features.
Hypothesis 2: Features extracted from sound event signals, including psychoacoustic features and features extracted from spatial filtering, can provide an effective two-stage classification of sound events that combines supervised and unsupervised classification methods.
Hypothesis 3: “Advanced Noise Map” can be used to plan and optimize noise mitigation strategies, resulting in a significant reduction of noise annoyance, sleep disturbance, and health risks for humans.
Hypothesis 4: Algorithms for unsupervised classification, in combination with algorithms for optimization of the number of clusters/classes (such as the elbow method, the silhouette optimization, the gap statistic, and the Davies-Bouldin index), can be used to accurately assess the number and diversity of biological sound events within the network of microphone arrays placed in natural environments, and to provide valuable information for the assessment of biodiversity.
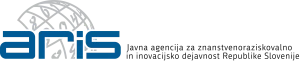
Šifra projekta: J7-50042
Veda: Interdisciplinarne raziskave
SICRIS: Spremljanje urbanega hrupa in biodiverzitete za zeleno prihodnost z akustičnim IoT radarjem s klasifikacijo dogodkov na osnovi UI (cobiss.net)
PUBLISHED PAPERS
- MUROVEC, Jure, PREZELJ, Jurij, ĆIRIĆ, Dejan, MILIVOJČEVIĆ, Marko. Zero crossing signature : a time-domain method applied to diesel and gasoline vehicle classification. IEEE sensors journal. 2025, vol. 25, iss. 3, str. 5128-5138, ilustr. ISSN 1558-1748. https://ieeexplore.ieee.org/document/10810281, Repozitorij Univerze v Ljubljani – RUL, DOI: 10.1109/JSEN.2024.3516876. [COBISS.SI-ID 221243907]
- PREZELJ, Jurij. Microphone arrays for localization of biological and anthropogenic sound events. V: Proceedings. 11th International Conference IcETRAN 2024, Niš, Serbia, June 03‐06, 2024. [S. l.]: IEEE, 2024. Str. [1-11], ilustr. ISBN 979-8-3503-8699-8. https://ieeexplore.ieee.org/stamp/stamp.jsp?tp=&arnumber=10645159, DOI: 10.1109/IcETRAN62308.2024.10645159. [COBISS.SI-ID 214759427]
- HVASTJA, Andrej, MUROVEC, Jure, PREZELJ, Jurij. Influence of biological sounds on long-term measurements of wind turbine noise. V: Proceedings. 11th IcETRAN papers in the IEEE Xplore, September 6, 2024. [S. l.]: IcETRAN, 2024. Str. 3-8, ilustr. https://www.etran.rs/2024/E_ZBORNIK_IcETRAN_2024/001_AKI1.3.pdf. [COBISS.SI-ID 214763779]
- TROST, Andrej, ŽEMVA, Andrej. Preizkušanje modelov vezij za digitalno obdelavo signalov. V: ŽEMVA, Andrej (ur.), TROST, Andrej (ur.). Zbornik triintridesete mednarodne Elektrotehniške in računalniške konference ERK 2024 = Proceedings of the 33rd International Electrotechnical and Computer Science Conference ERK 2024 : Portorož, Slovenija, 26. – 27. september 2024. Ljubljana: Slovenska sekcija IEEE: Fakulteta za elektrotehniko, 2024. Str. 12-15, ilustr. Zbornik … Elektrotehniške in računalniške konference (Online), 33. ISSN 2591-0442. https://erk.fe.uni-lj.si/2024/papers/trost(preizkusanje_modelov).pdf. [COBISS.SI-ID 212854531]
- ŠPEH, Jure, TROST, Andrej, ZADNIK, Damjan. Zajem podatkov akustične kamere na vgrajenem sistemu preko serijskega vmesnika. V: ŽEMVA, Andrej (ur.), TROST, Andrej (ur.). Zbornik triintridesete mednarodne Elektrotehniške in računalniške konference ERK 2024 = Proceedings of the 33rd International Electrotechnical and Computer Science Conference ERK 2024 : Portorož, Slovenija, 26. – 27. september 2024. Ljubljana: Slovenska sekcija IEEE: Fakulteta za elektrotehniko, 2024. Str. 8-11, ilustr. Zbornik … Elektrotehniške in računalniške konference (Online), 33. ISSN 2591-0442. https://erk.fe.uni-lj.si/2024/papers/speh(zajem_podatkov).pdf. [COBISS.SI-ID 212852483]
- ZADRAVEC, Aljaž, PEČAR, Borut, VRTAČNIK, Danilo, MOŽEK, Matej. Realtime data acquisition and processing in distributed wireless sensor networks. V: LIPOVŠEK, Benjamin (ur.), JANKOVEC, Marko (ur.). 59th International Conference on Microelectronics, Devices and Materials & Workshop on Electromagnetic Compatibility: From Theory to Practice : conference 2024 : proceedings : October 2-October 4, Rimske Toplice, Slovenia. Ljubljana: MIDEM, Society for Microelectronics, Electronic Components and Materials, 2024. Str. 92-97, ilustr. ISBN 978-961-95495-3-7. [COBISS.SI-ID 211649795]
- HVASTJA, Andrej, ĆIRIĆ, Dejan, MILIVOJČEVIĆ, Marko, PREZELJ, Jurij. Assessing Air and Noise Pollution Through Acoustic Classification of Vehicles Fuel Types Using Deep Learning, accepted for publication in Heliyon, 27 Feb 2025, https://authors.elsevier.com/tracking/article/details.do?aid=43062&jid=HLY&surname=Hvastja
PAPERS UNDER REVIEW (March 2024)
- MUROVEC, Jure, HVASTJA, Andrej CERKOVNIK Nejc PREZELJ Jurij, Application of Spectral Immission Directivity for Long-term Environmental Noise Measurements, Journal: Applied Acoustics,
- PREZELJ Jurij, HVASTJA Andrej, MUROVEC, Jure, ČUROVIČ Luka, Insect Sounds as Background Noise during Measurements of Wind Turbine Sound Power, Journal: Heliyon
DEVELOPED HARDWARE
Figure 1: Improved version of MEMS microphone array
EXPERIMENTAL RESULTS
By analyzing the direction from which sound originates in sub-windowed time intervals, we can accurately pinpoint sound sources using simple triangulation methods. With only two synchronized measurement systems—and provided the sound source is within a range that ensures adequate detection resolution—precise localization is achievable for each sound event segment. This approach enables the determination of sound source positions with nearly arbitrary temporal resolution. In our experimental setup, measurements were conducted using a 50-millisecond time constant, demonstrating both the feasibility and accuracy of our triangulation-based localization method.
Measurements were performed in a residential area where the noise reaching the microphone antennas was often primarily reflected from the facades of nearby buildings. Using our approach, we successfully localized sound sources within the defined area, provided that the conditions for the angles of sound arrival at the individual microphone antennas were met.
An example of the results is presented in Figure 1. Figure 1A shows the sound pressure level (SPL) as a function of time, with an integration period of 50 milliseconds measured by a single microphone. Background noise levels were around 40 dB, bird sounds reached approximately 55 dB, and anthropogenic noise—specifically from a lawn mower—exceeded 55 dB. Figure 1B illustrates the direction of arrival for a sound event as captured by two microphone arrays. For each measured SPL, a corresponding direction was assigned, ranging from –π/2 to +π/2, derived from two microphone arrays (blue dots for Array 1 and red dots for Array 2), as depicted in Figure 1B. Converting these directions into a polar plot, where the radius represents the SPL at a given time, produced the plot shown in Figure 1C. These polar plots are valuable not only for further sound source localization (SSL) but also as features for sound event classification; each point indicates the identified direction of a sound event (angle) and its corresponding SPL (distance from the center) within a 50-millisecond window.
Figure 1: Scatter diagram in polar coordinates indicating direction of sound event arrival (angle) and sound event SPL (radius). Each point represents a detected sound event within 50 msec, and its direction of arrival.
Based on the results in Figure 1, a noise event source map was generated (Figure 2) by placing a circle on the map at the center of each detected sound location every 50 milliseconds. The radius of each circle is proportional to the sound pressure level recorded during that interval, and the circle’s color varies with the level: a small blue circle represents a low noise level of around 40 dBA, typically originating near the coordinate system’s origin, whereas a larger red circle denotes a higher noise level of approximately 65 dBA. Figure 2 overlays the measurement location map with the detected sound source positions. Results are presented for two distinct periods: one during which a lawn mower was in operation and another when birds were actively singing. The bird data, indicating their presence in the trees, were corroborated by visual observations during the recordings. For clarity, the results are also shown schematically.
Figure 2: Sound source map based on a scatter plot from sub-windowed triangulation. The map overlays sound sources with a 50 msec time resolution, with schematic representations for grass mowing (left) and birds in trees (right).